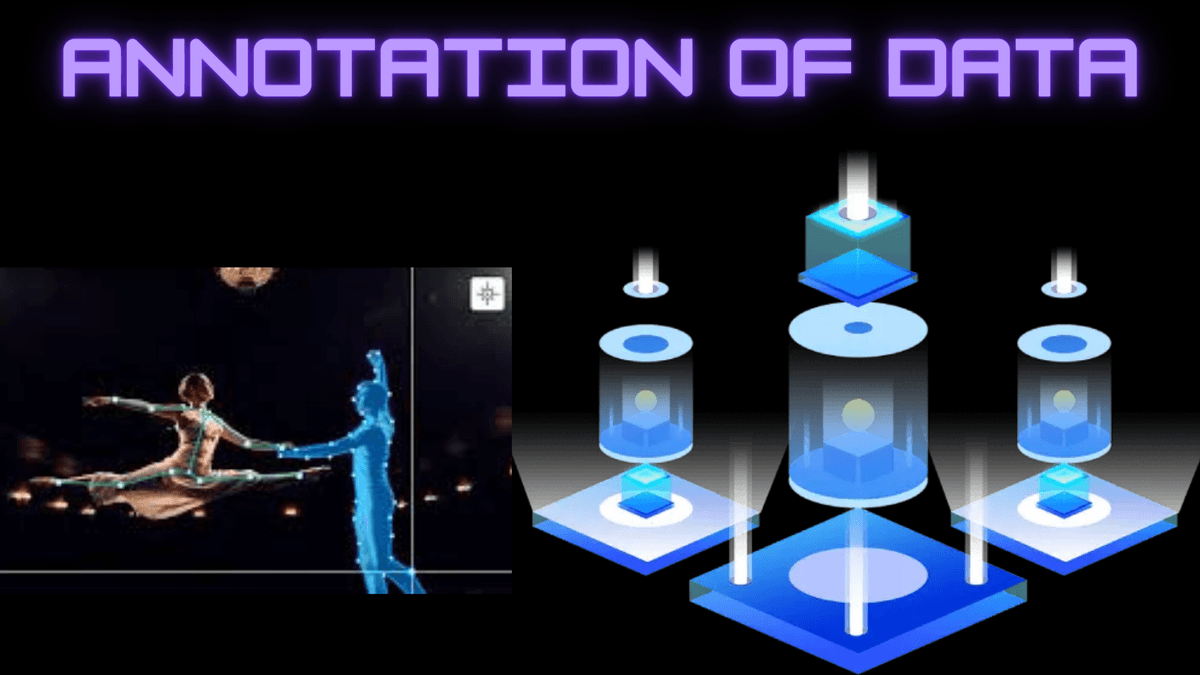
What are DATA ANNOTATION SERVICES?
You want to provide large quantities of raw data to artificial intelligence (AI) robots so they can do activities like those performed by humans. The problem: These machines can only act following the parameters you Specified for the data set. Data annotation is the primary technique for bridging the gap between example data and AI/machine learning. Data annotation is adding categories, labels, and other contextual aspects to a raw data set so that computers can interpret and act on the information. The use of data annotation in applications and some of the present and foreseeable advantages of the technique are covered in more detail below. The strength of machine learning initiatives, in general, is data. Your conclusion will be more accurate the more data you have.
Raw data alone, though, is insufficient. For the machine learning system to accurately identify objects in a given image, grasp spoken language, and do several other tasks, you must annotate this data. A raw data set is "annotated" with categories, labels, and other contextual elements so that computers can understand and use the information. Before a product is released, its accuracy should be increased. In other words, human data annotations will need to manually go over each image to determine whether the quality of the annotations is good enough to train the algorithms.
What are the principal of DATA ANNOTATION SERVICES?
• Costing of annotation data may be carried out manually, automatically, or both. However, manually annotating data is time-consuming, and must maintain the quality of the data.
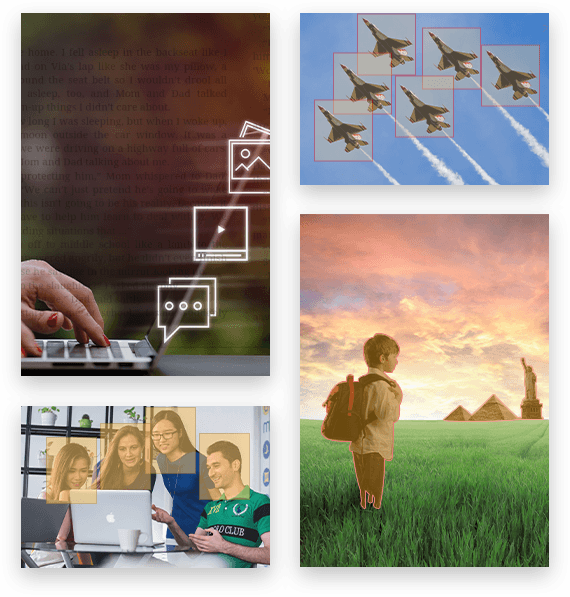
• Accuracy of Annotations: Poor data quality can be caused by human mistakes, which directly impacts how well AI/ML models estimate the future. A Gartner study found that poor data quality costs companies 15% of their revenue.
Below, we go into greater depth on how Data Annotation Services are used in applications and some of the current and future benefits of the method. Data is the main asset of machine learning projects in general. The more information you have, your ultimate decision will be more accurate. However, raw data alone is insufficient. You must annotate this data for the machine learning system to recognize items in a given image, comprehend spoken language, and do several other tasks.
Sentiment annotation: In this technique, a human annotation gathers training text for AI while highlighting words and phrases' arbitrary and emotional implications. Sentiment annotation helps AI comprehend the underlying meaning of the text.
Intent: Similar to sentiment annotation, intent annotation focuses on designating the ultimate objective of the user or the human intent underlying particular remarks. In the context of customer support, where AI-powered chat bots must comprehend the specific data or outcomes they should present to a human user, intent annotation offers expertise.
Semantic Annotation: Semantic Annotation is driven by buyer-seller relationships and strives to improve the precision of product listing labels so that artificial intelligence (AI) can suggest or create exactly what people are looking for in search results.
Image Analysis
Image annotation's most basic goal is to provide information about images, keywords, and descriptors in contrast to other aspects of the images. Thanks to Image Annotation, screen reader users can examine images, and it also helps websites like stock image aggregators discover and distribute the appropriate photos in answer to user queries. Contextual annotations have been added to detailed images of streets and people's bodies over time, improving AI capabilities. These pictures are practice data for autonomous vehicles and medical diagnostic equipment.
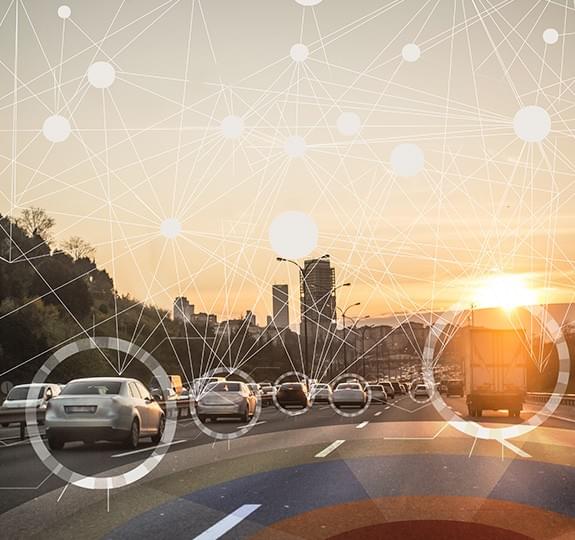
Text classification
Depending on the subject, text categorization assigns classifications to certain words inside a document or a complete paragraph. Users can easily find the information they require on the website.
Audio Annotations
Many mobile and Internet of Things (IoT) devices rely on voice recognition and other audio comprehension features. However, they can only learn the meanings of sounds after frequent audio annotation. With speech and other sound effects as their starting point, audio annotators name and categorize audio recordings based on things like intonation, dialect, pronunciation, among other things, and volume. Home assistants and other devices rely on audio annotation for speech and audio recognition.
YouTube commentary
Video annotation combines several images and audio annotation characteristics to aid artificial intelligence (AI) in determining the importance of the sound and visual elements in a video clip. Video annotation has become increasingly important as technologies like self-driving cars and home devices have.
Advantages Of DATA ANNOTATION SERVICES
- Data with annotations Because it is a facilitator rather than the finished product in the development of reliable AI, its advantages may be related to improving the efficiency and dependability of the AI engine. That serves as both its primary advantage and goal. Its advantages are inextricably linked to those of AI.
- Regarding data output, the GIGO (Garbage In, Garbage Out) principle frequently leads to issues. The output quality of a machine or computer can only be as good or bad as the input data it took in and processed.
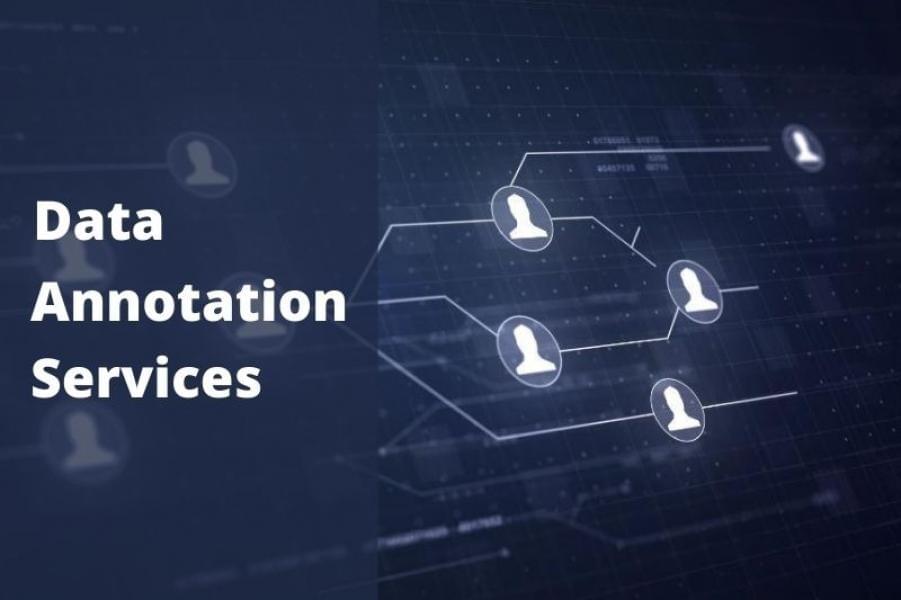
- While terrible input data can never yield good outputs, good input data can nevertheless be damaged by a computer program or human error. As a result, the main benefit of data annotation is that it finally leads to creation of a trustworthy and clever AI engine.
Data Annotation Services offered by GTS.AI
GTS.AI provides annotation services that are crucial to the functioning of supervised learning models since the type and quantity of annotated data determines their efficacy and correctness. Annotated data is vital because Finding high-quality Dataset For Machine Learning is one of the challenges GTwayS.AI has accepted and done in effective way. Machine learning models have many varied and essential applications.