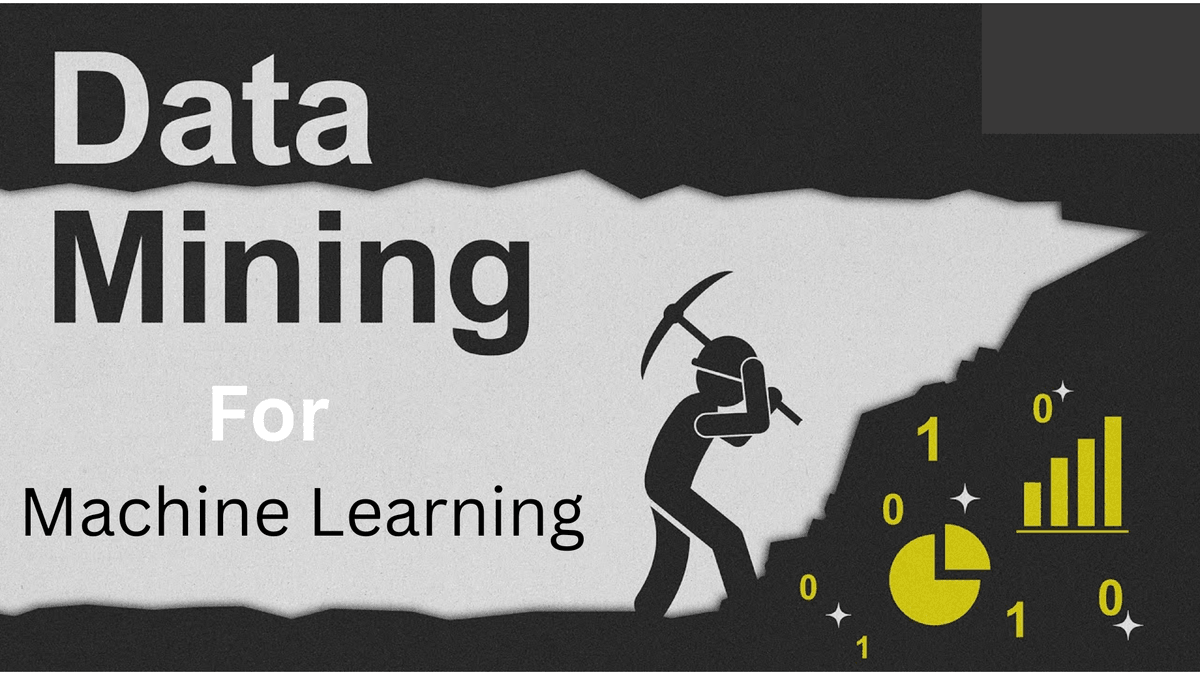
Introduction
Data mining is extracting useful information from a huge amount of data. It is used to locate new, accurate patterns that are useful inside the data, allowing one to obtain pertinent information about the organization or person who needs it. One person uses the tool. Individual's use.
What Is Machine Learning?
The process of identifying algorithms that have got better thanks to data-based knowledge is known as machine learning. Machines can learn without human involvement due to algorithms designed by studying, analyzing, and developing. It is a tool to improve the efficiency of machines by eliminating the need for humans.
Data Mining Process:
- Data Cleaning
- Data Integration
- Data Reduction
- Data Transformation
- Data Mining
- Pattern Evaluation
- Knowledge Representation
Mining raw Dataset for Machine Learning:
Bring the matter to the forefront as soon as possible.
You can decide which information is most beneficial to gather by understanding what you wish to predict. Data exploration and a determination to think about the problem in terms of classification, clustering or regression, and ranking should be considered while framing the issue.
The most difficult part of the task could be convincing an organization to embrace a data-driven mindset. Fighting data fragmentation is the first step in using ML to perform predictive analytics. Different departments and even various departments' tracking points can create data silos. Although marketers have access to CRM, the clients still need to link the web's analytics. If you've got multiple ways to engage, acquire, and retain, it's often only possible to integrate some data streams into one database; however, it's generally possible to manage it.
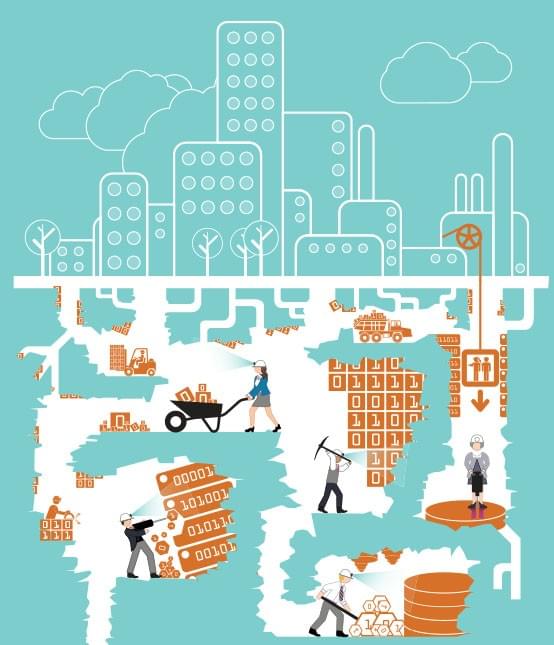
Collecting data is typically carried out by an engineer in data, the person responsible for building Dataset For Machine Learning infrastructures.
Data Warehouses and ETL
- Data Lakes and ELT
- Handling human factors
- Examine the quality of the data you have.
Are you confident in your information? It is the first thing you should ask. Lack of data makes it difficult for the most sophisticated algorithmic machine-learning algorithms to perform their job.
Things to consider
- How real is human error?
- Did you encounter any technical issues while transferring data?
- How many values that are omitted do your records have?
- Are your files up for your job?
- Is your data imbalanced?
Formatting of data to guarantee the consistency of data
The format that you're using is a different name for data formatting. Converting a data set into the best format for your machine learning software is relatively easy. Ensuring all variables in an attribute are written consistently is essential if you're using various Image and Video Dataset from different sources or if various people manually modify your database.
Data reduction
Because of the huge amount of data available, the temptation is to integrate the most information possible. It is not a good idea. You indeed want to collect as much data as you possibly can. But, it's best to reduce data when you're building a data set with a particular task in your mind. Common sense will help guide you once you're aware of the property you are targeting (the value you want to predict). With no forecasting tools, it is possible to determine the most important variables, which will add to the complexity and size of the collection.
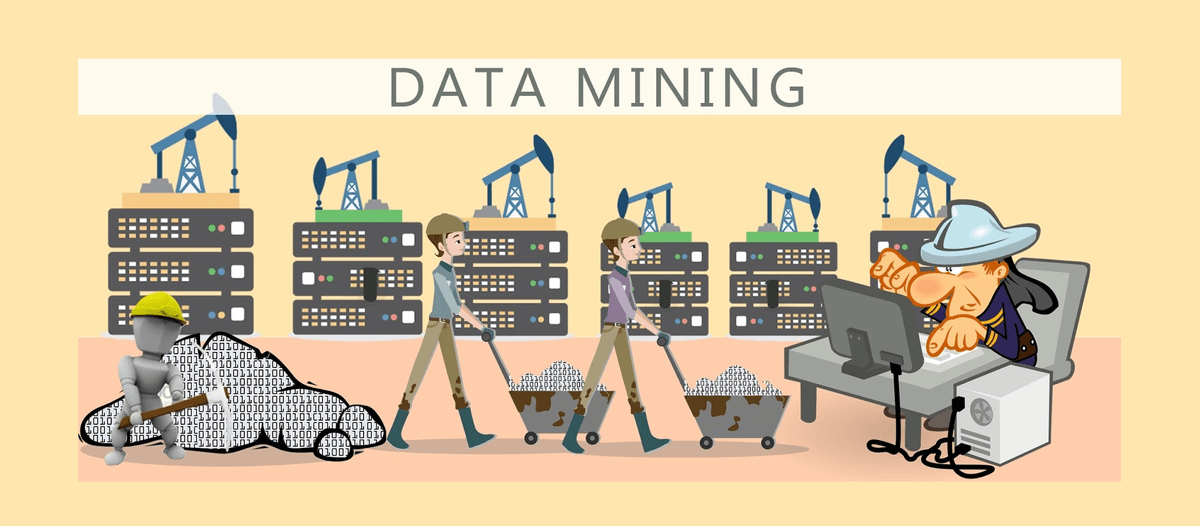
Complete cleaning of data
It is a must, as missing values can make predictions less precise. Approximate or assumed values tend to be "more appropriate" for an algorithm in machine learning rather than missing values. There are methods to "better presume" which value is missing or circumvent the issue even if you do not know the exact value. What is the best way to purge data? The information and the domain you own play an important influence in determining the optimal method of action.
Combing attributes and transactional data
Information about attributes, like the demographics of a user or their age, is more amorphous and doesn't have any obvious connection to particular events.
Data scaling
Data rescaling can be described as a kind of data normalization that aims to improve a data set's quality by reducing its size and preventing an environment in which certain values are underrepresented compared to others.
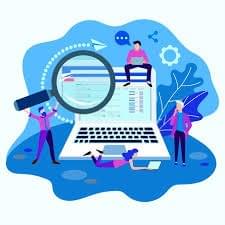
Disambiguate data
Sometimes, converting numbers into categorical values helps you make predictions more accurately. It can be done, for example, by categorizing the entire spectrum of values.
GTS.AI Organizes Dataset For Machine Learning Projects
We at Global Technology Solutions (GTS.AI) provide all kinds of data collection such as Image Data collection, Video Dataset, Speech Data collection, and text dataset along with audio transcription and Video Annotation Services. Do you intend to outsource image dataset tasks? Then get in touch with Global Technology Solutions, your one-stop shop for AI data gathering and annotation services for your AI and ML.