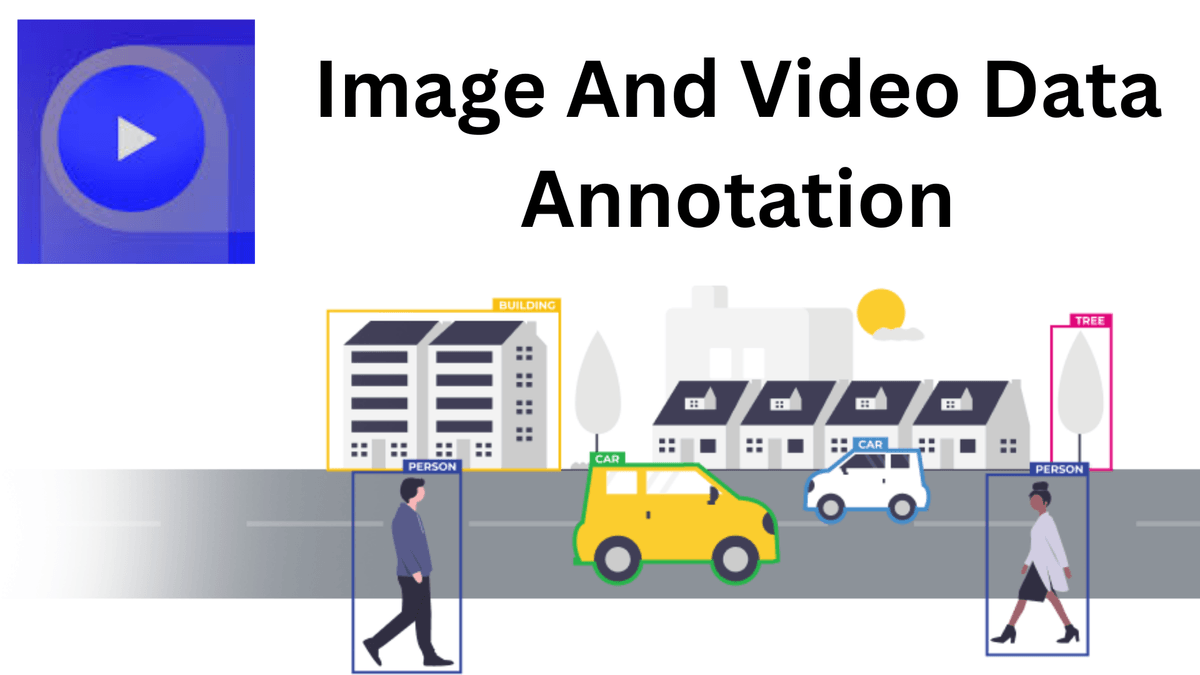
Introduction
Machine learning has enabled Al to become more sophisticated and more sophisticated. Autonomous vehicles such as facial recognition, autonomous drones, health software, applications for imaging medical and military strategy all have relied on deep learning, which has resulted in stunning technological advances.
The main ingredient in getting this to take place can be traced to Data Annotation Services. By adding metadata to datasets, you can recognize patterns and helps models to recognize objects.
The Confrontation of Pixel Perfect Semantic Segmentation
Image annotation doesn't come without problems. Consider the huge amount of information required to allow autonomous vehicles to function. Cameras need to be able to detect and classify objects immediately. One of the major challenges for self-driving cars is the incorrect classification of things. Only a few pixels can cause a pedestrian to be confused with the light pole, and failing to be able to predict its movement.
It's supervised machine-learning that teaches autonomous vehicles how to identify objects. But it's only as effective as the training datasets with labels which are used as used as input. If you have to deal with thousands of million of points of information, it's is a daunting job. In the past there were just two options to choose the data points from videos.
- Select some frames or images, and you will possess a little bit of information
- Make sure to annotate the frames in order to collect more information
Utilizing too many information points could result in inaccurate data , and noting additional frames takes more resources and is a costly, labor-intensive long process. Annotating video frames frame-by-frame is an extremely time-consuming way. In order to make annotations research, most researchers use boundaries boxes, or polygons tools. Bounding boxes usually contain background pixels and add noise to the dataset used for training. Noise is interpreted in various ways. Furthermore, poor specification documentation could lead to an unclear interpretations, which could result in inconsistencies among the data that is collected.
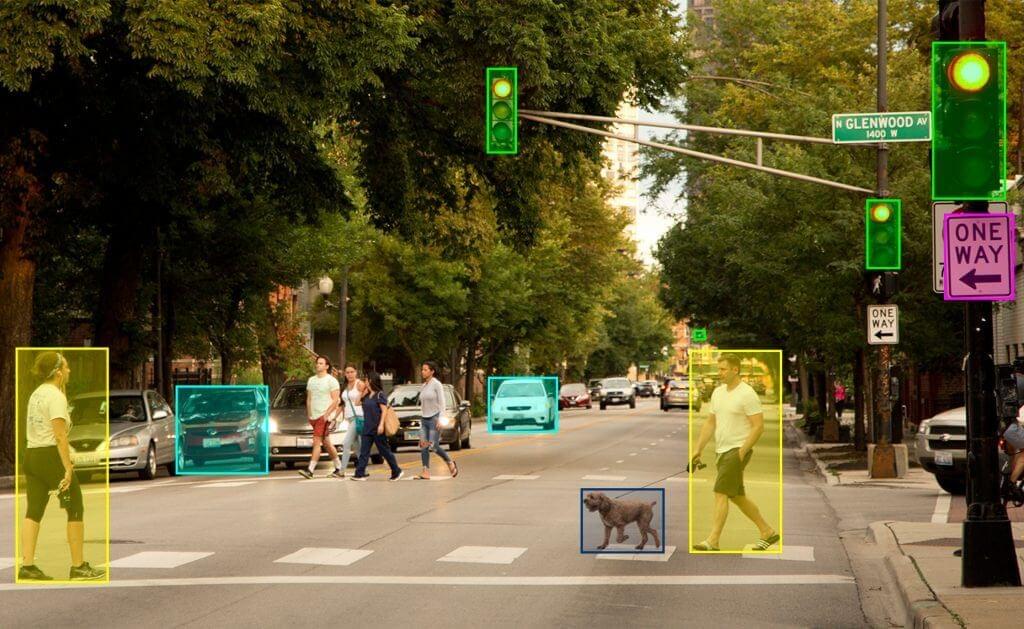
What Is Pixel Perfect Semantic Segmentation?
The majority of methods for image annotation utilize bounding boxes. They can also be adapted to objects detectors in order to produce segments. Pixel Perfect Segmentation assigns every pixel a map and assigns an object's class and an instance identification label for each one. With the present technology for annotations, experts are required to go frame-by-frame in order to paint the data of objects. For accuracy, researchers could have to classify and identify objects each frame in order to gather the information needed. If you realize that a single minute of video has many thousand frames, you will quickly observe how difficult this job becomes.
Pixel-perfect Semantic Segmentation to Artificial Intelligence-Assisted Data Annotation tools require fewer frames to be manually annotated. It is the GTS exclusive ML model tracks objects and interpolates their positions between frames, without compromising accuracy. Researchers have a vast understanding of the entire video of 10 minutes using a single segmentation of the first frame. This can result in a 10-fold increase in the number of labels while reducing the time for labeling to minutes. As time passes the algorithm gets smarter and more efficient to reduce the amount of frames required.
It's smart enough to detect the movement of objects and then automate the process. If it loses an object like when it disappears from the display, it will know to stop. By separating objects down to the level of the pixel The algorithm produces more data , making tracking much easier. Machine learning helps make Al Al better at analyzing Dataset For Machine Learning as more information is consumed, while the Al algorithm performs much work needed to keep track of objects as they move. This leads to a significant reduction in the amount of frames that require hand-written annotation without affecting the quality of the information that is gathered.
The Benefits of AI-Assisted Pixel Perfect Segmentation
The improvement in data results in deeper insights, better training, and improved performance. The best collaborative platform will assist data researchers, data analysts, algorithm developers and data engineers to manage the entire process with ease. The benefits include:
- Elimination of labeling repetitive tasks
- Transform raw data into labeled data sets
- Reduce error space around objects
- Consolidate labels across multiple annotations on the same image.
- Eliminate data-distribution biases
- Enhance the diversity of data
- Build training datasets with efficiency and enough complexity to provide better results
Pixel-wise annotation of video leads to more reliable dataset and deeper insights , meaning that the trial and error process of designing models can be averted with a deeper understanding.
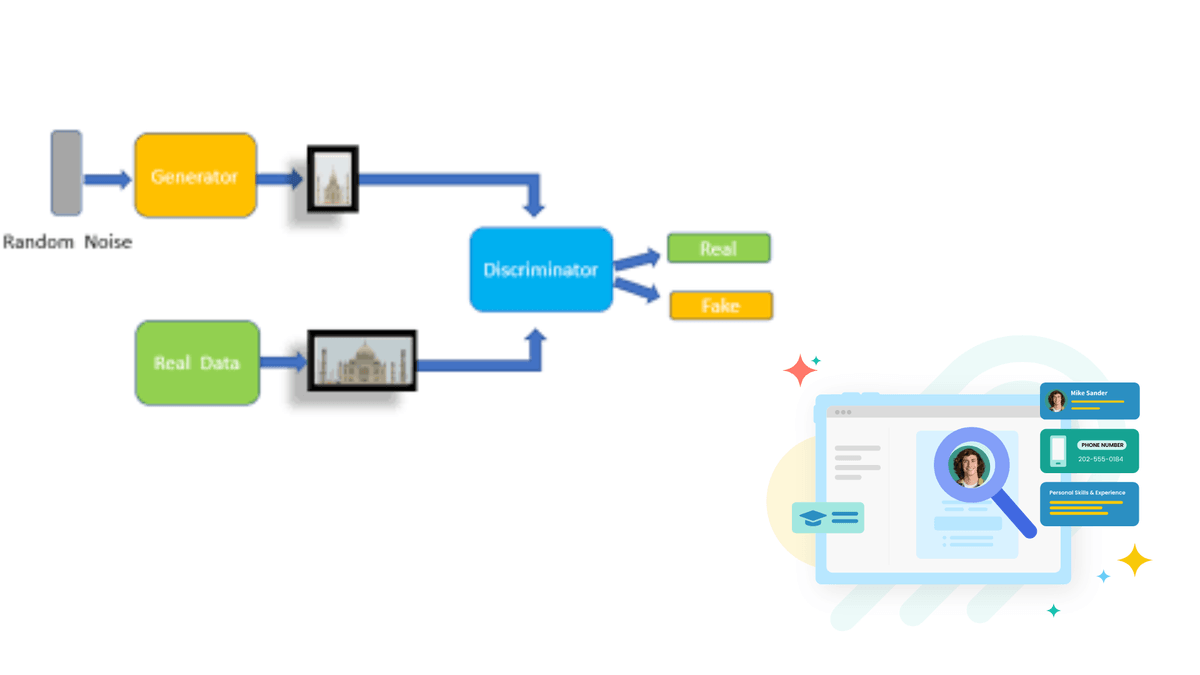
Richer Datasets With GTS
The demand to mine and extract meaningful patterns from massive data sets will only increase. As technology improves and tools for segmentation and Al are transforming the market. The market for annotations worldwide is currently worth at $209 million, and increasing at the rate of 15.8 percent per year. With the need for greater and more data grows and this rapid growth is expected, it requires better methods of collecting and annotation for larger, better and more extensive datasets.
Global Technology Solutions (GTS) provides comprehensive computer vision solutions by giving Annotation Service along with, OCR Training Dataset and Audio Transcription Services to diverse industries including security and surveillance industrial, transportation smart cities, pharmaceuticals, and consumer electronics through the entire lifecycle of a model, including algorithm selection, learning and validation, through inferencing, deployment and maintenance.