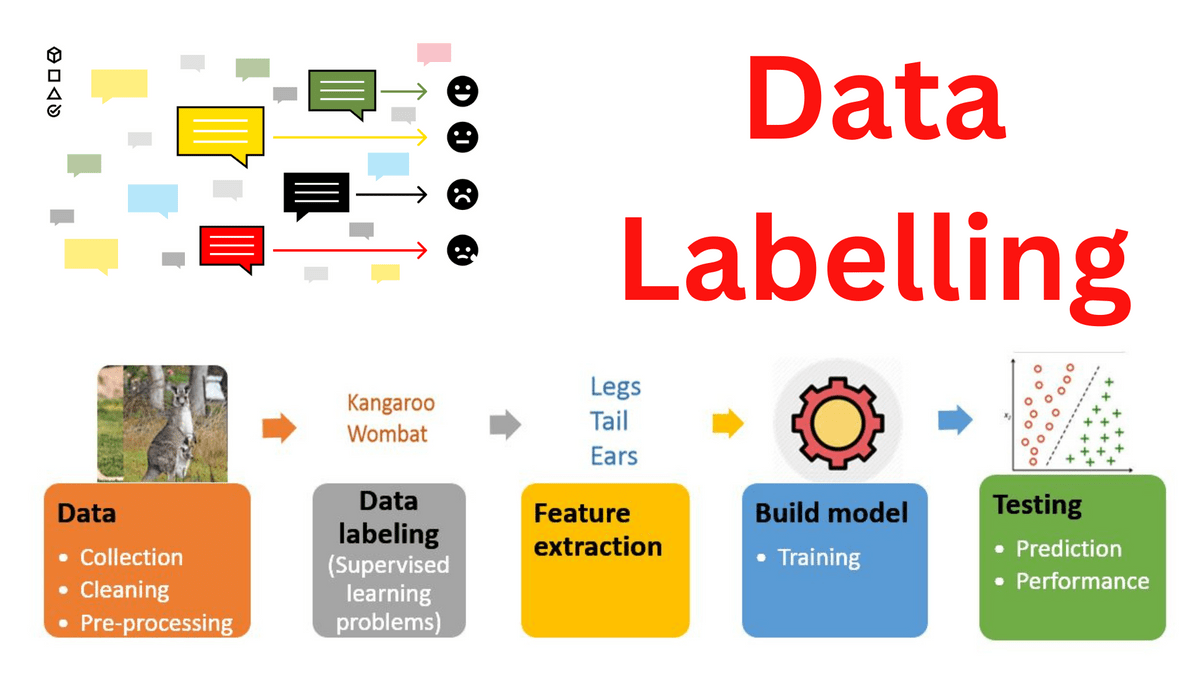
What is Data Labelling?
Data Labelling — or information explanation — alludes to the act of adding labels or marks to crude data like photos, recordings, text, and sound.
These labels depict the information’s substance type, alluding to different properties and attributes of the data of interest. This permits an AI model to figure out how to perceive that kind of article when it’s met in information without a name. To prepare computer based intelligence and AI calculations to comprehend and gain from your information, you really want a very much smoothed out and great course of information marking.
Your marking must be basically as exact as could be expected, whether it’s named by class, subject, topic, or some other classification. With complete information marking, the artificial intelligence framework performs better and conveys more exact outcomes.
Most normal kinds of Data Labelling
Information can be organized or unstructured. Organized information is ordinarily quantitative and numbers-based, while unstructured information is commonly subjective and can’t be investigated utilizing regular information examination apparatuses.
Data Labeling Company are responsible for various kinds of information with the assistance of various man-made intelligence sub-innovations.
PC Vision for pictures
PC Vision (CV) is a computer based intelligence subset that permits machines to perceive objects in a picture. This implies that PCs would be able “see” what’s in the picture and name it, very much like the natural eye would however without a similar time speculation.
To do this, PCs need:
Picture comment: The most common way of adding names to pictures.
Video comment: The most common way of adding names to recordings.
At the point when we make a computerized border around things in a picture for PC Vision — otherwise called a jumping box — the PC can isolate the various segments of the image for order.
This preparing information can then be utilized to make a PC Vision model that is ready to sort pictures, perceive the place of items, recognize significant spots in a picture, and part a picture. For instance, numerous web based business stores have carried out PC Vision instruments to perceive objects on item pictures and label them. These labels help site guests effectively find what they’re searching for. Suppose you have an internet based design store. A PC Vision instrument can handle large number of your item pictures containing style things. In the event that there’s a red skirt on the picture, the device will add labels like red, velvet, A-line, creased, and so on. With these labels added, any individual who comes to the page and looks for a “red velvet skirt” will actually want to effectively find what they’re searching for.
No code PC Vision permits individuals of all degrees of specialized information to work on the productivity of their business tasks. Utilizing PC Vision, you can name your picture and video data in a straightforward mechanized process as opposed to employing somebody with a profoundly qualified range of abilities to construct an in-house arrangement.
Natural Language Processing (NLP) for text
Natural Language Processing (NLP), is a part of Man-made brainpower that permits PCs to grasp human discourse. NLP consolidates dialects, measurements, and AI to research the structure and laws of language to foster clever frameworks that can grasp text and discourse. Basically, you are helping the machine to fathom language.
Natural Language Processing is the innovation used to help PCs to grasp normal human language. To make your preparation dataset for NLP, you should first physically pick applicable lumps of text or label the text with specific names. Feeling Investigation and Named Substance Acknowledgment (NER) is finished utilizing NLP models.
NLP can assist you with computerizing business cycles and gain noteworthy experiences from them. Any text-related cycle can be utilized in NLP, like online entertainment examination.
For what reason is Data labelling significant for Machine Learning?
Information naming is significant for man-made intelligence since it helps train your model to comprehend and sort approaching information. Information marking permits PCs to precisely get a handle on genuine settings, which opens up new potential for a large number of enterprises. Think about the accompanying situation: you wish to prepare an Opinion Examination model. To do this, you should give the artificial intelligence model examples of positive, pessimistic, and nonpartisan feelings that have been classified so it can start to separate between the three. You’ll likewise have to add expresses that mirror the regular human language, like mockery, humor, and incongruity. Assuming your names are mistaken or vague, your computer based intelligence model’s expectation will be straightforwardly impacted by this. That is the reason it’s vital to ensure you have an adequate number of picture and Video Dataset of interest and that they are named accurately prior to robotizing a cycle with man-made intelligence. The nature of the preparation information decides the progress of your man-made intelligence model — it should be significant and designated at what you’re hoping to comprehend. Whenever you’ve coordinated your preparation information and marks, you’ll have the option to utilize them to make your regular exercises simpler.
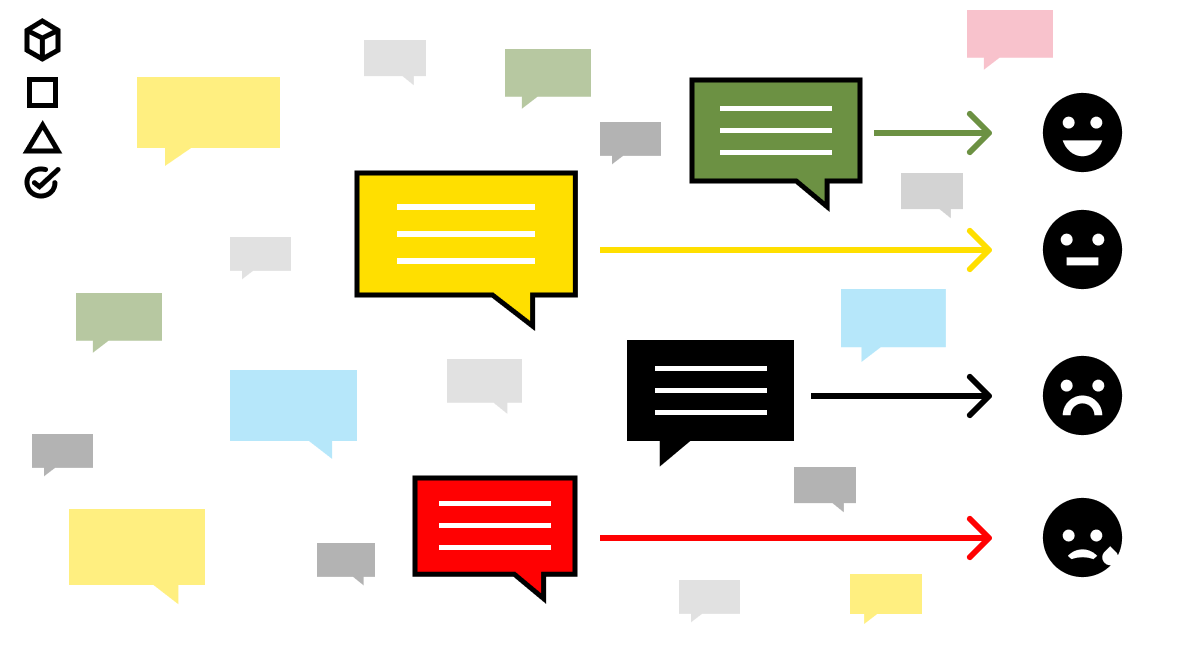
Think about the accompanying situation: you wish to prepare a Feeling Examination model. To do this, you should give the man-made intelligence model occasions of positive, pessimistic, and nonpartisan feelings that have been classified so it can start to separate between the three. You’ll likewise have to add expresses that mirror the normal human language, like mockery, humor, and incongruity. Assuming your names are off base or vague, your artificial intelligence model’s expectation will be straightforwardly impacted by this. That is the reason it’s critical to ensure you have an adequate number of data of interest and that they are marked accurately prior to mechanizing a cycle with man-made intelligence. The nature of the preparation information decides the progress of your man-made intelligence model — it should be significant and focused on at what you’re hoping to comprehend. Whenever you’ve coordinated your preparation information and marks, you’ll have the option to utilize them to make your ordinary exercises more straightforward.
Labeled versus Unlabeled Data
An information point that contains a tag, like a name, a sort, or a number, is alluded to as marked information. Information that hasn’t been doled out a mark is alluded to as unlabeled information. To comprehend the contrast between marked information and unlabeled information, we’ll go through the three kinds of AI that we can utilize. Each sort of AI requires an alternate kind of information.
Managed AI
This sort of AI requires marked information to learn. Managed learning models are prepared with marked information and afterward used to figure future results. This preparing dataset contains the two information sources (the data of interest, e.g.: picture) and results (the mark, e.g.: front room). These assist the model with working on over the long run by realizing the very information focuses that come in and the data that ought to emerge accordingly (the name).
Regulated learning can do grouping, meaning it can sort information into classifications (e.g.: is this a vehicle, bicycle, or train?), and relapse, which alludes to its capacity to find ceaseless relationships amongst reliant and free factors (e.g.: in view of information, foreseeing the expense of a house in light of a picture of it). Anticipating land costs or naming land pictures are instances of administered learning. For a calculation to have the option to foresee this, it needs current and past costs. It likewise needs information about the quantity of rooms, size, the year it was assembled, etc. The outcome is a forecast of the house cost in view of past and current information.
Solo AI
Solo learning requires unlabeled information. The model runs without monitoring any names that the information might contain. This learning strategy is reasonable for issues where we have practically zero clue about what our outcomes ought to resemble. These calculations uncover stowed away examples or information bunches. It’s the most ideal choice for exploratory information investigation, strategically pitching methods, purchaser division, and picture ID due to its ability to recognize likenesses and differences with no human guidance.
Semi-regulated AI
Semi-regulated AI requires a blend of marked and unlabeled information. It directs the classification and extraction of highlights from a bigger, unlabeled informational collection utilizing a more modest named informational index during preparing. Set forth plainly, semi-directed learning involves named information as an illustration to label the unlabeled information.
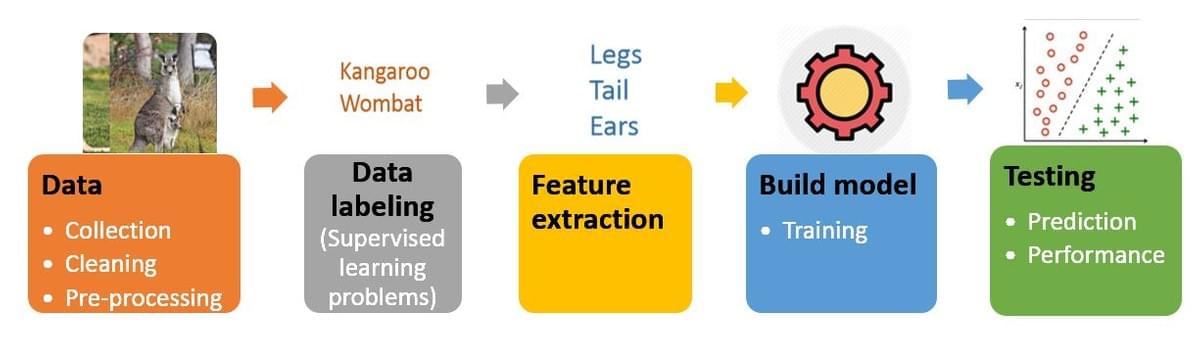
Data Labelling With GTS Services
We at Global Technology Solutions (GTS) provide all kinds of data collection such as Image Data collection, Video data collection, Speech Data collection, and text dataset along with audio transcription and Data Annotation Services. Do you intend to outsource image dataset tasks? Then get in touch with Global Technology Solutions, your one-stop shop for AI data gathering and annotation services for your AI and ML.